I recently had the pleasure of speaking with Mike Moran about implementing AI in B2B marketing. Our conversation couldn’t have been more timely. Small marketing teams across the tech landscape are feeling the mounting pressure to adopt AI solutions — not only to stay competitive, but also to cut costs while still driving growth.
Here’s the challenge: if your primary goal with AI is cutting costs, you might be setting yourself up for disappointment. At New North, we’ve seen this play out time and again with our B2B tech clients. As Mike pointedly observed during our discussion, “If you set out to reduce costs, you are unlikely to make your marketing more effective.”
This insight gets to the core of the AI imperative. While the promise of doing more with less is appealing—especially for small B2B tech marketing teams with limited resources—the most effective approach challenges conventional wisdom and flips it on its head.
In this article, I’ll share a clear roadmap for results-focused AI implementation based on my conversation with Mike and our experience working with tech companies like yours.
Let me be clear about my commitment to you: no fluffy buzzwords, no theoretical frameworks too complex to implement, and no strategies that require a data science degree. Just practical approaches that your small marketing team can put into action right away.
Setting the Right Foundation: Understanding Effectiveness vs. Efficiency
In my years hosting Content Logistics, I’ve noticed how often effectiveness and efficiency get conflated in marketing discussions. Let’s clarify this critical distinction before diving into AI implementation.
- Effectiveness measures whether your efforts achieve their intended outcome. Are you reaching the right audience? Are they taking the desired actions? Is your message resonating in a way that drives business results?
- Efficiency is about resource optimization. How quickly can you produce that content? How many channels can you manage with your current team? Can you reduce costs while maintaining output?
Both matter tremendously, but the order of operations is crucial. Focusing on effectiveness first consistently delivers better long-term results.
Mike shared a rather blunt analogy that stuck with me: “If I have the greatest project ever for you, which is to stop doing marketing. You get a 100% savings. It’s fantastic.” He paused for effect before adding, “But that’s probably not what you want.”
The point lands perfectly. Maximum efficiency with zero effectiveness is worthless. I experienced this firsthand when we helped a SaaS client overhaul their content approach. Previously, they’d implemented an AI tool that quadrupled their content output (impressive efficiency), but engagement metrics had plummeted because the content failed to address customers’ actual pain points.
When we shifted focus to effectiveness first, deeply understanding audience needs before scaling production, not only did engagement metrics recover, but the content creation process became more efficient as we eliminated wasteful efforts.
Take a moment to assess your current approach: Are you measuring success by output and cost reduction, or by customer engagement and business outcomes? Your answer reveals whether you’re prioritizing efficiency or effectiveness in your AI implementation journey.
Step 1: Establish The Right Metrics Before Implementing AI
The metrics you choose to track determine the success story you’ll be able to tell. From my conversations with CMOs and marketing directors at B2B tech companies, I’ve found that those struggling with AI implementation often track vanity metrics that look impressive in reports but don’t connect to business outcomes.
So, which metrics matter for B2B tech companies? Mike’s framework offers elegant simplicity: “Did people see your marketing? If they saw it, did they click on it? And then did they buy something?” This progression from awareness to engagement to conversion creates a solid foundation for meaningful measurement.
Consider a SaaS company tracking social media impressions without connecting those impressions to qualified leads or sales. While AI might help generate more impressions efficiently, it’s not moving the needle on revenue.
For small teams just starting this journey, I recommend a basic attribution model that follows these steps:
- Identify 3-5 key conversion points in your customer journey
- Track source attribution for each conversion (where did these leads come from?)
- Measure time-to-conversion for different channels
- Connect marketing touchpoints to actual revenue
With these metrics in place, you’ll have clear benchmarks to evaluate whether AI implementation is improving what truly matters.
Step 2: Collect and Analyze the Right Data
You don’t need a team of data scientists to make smart decisions based on marketing data. The key is collecting focused information that directly connects to those effectiveness metrics we established in Step 1.
Start by leveraging the data you already have. Many B2B tech companies already have untapped insights in their systems — they just don’t know where to look. During our conversation, Mike highlighted the tool Impact Hero from SEMrush as an affordable way to measure content effectiveness for teams without enterprise-level budgets.
A simple yet powerful tactic is to analyze what users are searching for on each page of your website. That will reveal precisely what information visitors want but aren’t finding — essentially telling you what content to create next.
In my work with B2B tech companies, I’ve consistently found that these existing data sources often reveal more actionable insights than complex analysis of broad datasets. The key is connecting these insights directly to your effectiveness metrics rather than getting lost in data for data’s sake.
Before investing in new data collection tools, examine these often-overlooked sources:
- Internal site search queries
- Support ticket common questions
- Sales call objections
- Email response rates by topic
These existing data points often reveal more actionable insights than sophisticated AI analysis of broad datasets.
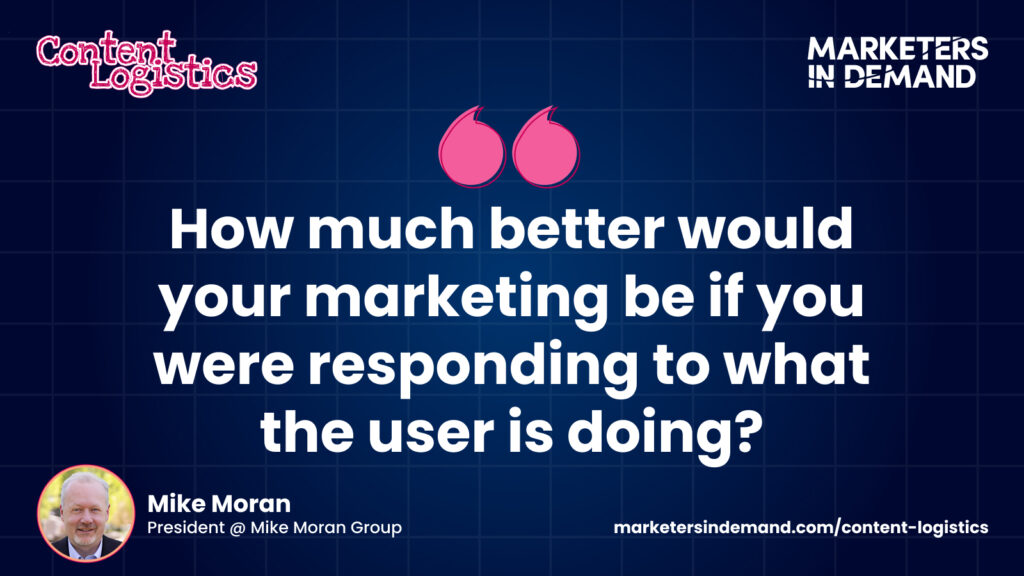
Step 3: Make Better Human Decisions First
Before automating any part of your marketing with AI, focus on improving your human decision-making process. The quality of AI output is directly proportional to the quality of human input and oversight.
A key principle to keep in mind is decision velocity—the idea that making faster decisions with reasonable confidence often drives better outcomes than waiting for perfect certainty. AI tools should accelerate this process, helping you move quickly and iterate effectively rather than replacing human judgment altogether.
At New North, we’ve found that AI excels at helping teams test multiple messaging variations, audience segments, and creative approaches simultaneously. The key is having humans set the strategic direction and interpret the results.
Decisions that should remain primarily human include:
- Setting strategic objectives
- Determining brand voice and positioning
- Interpreting data in the context of market conditions
- Building relationships with key accounts
When used thoughtfully, AI can significantly speed up tasks like research and outlining, freeing your team to focus on higher-value strategic decisions that still require a human touch.
Step 4: Implement Simple Attribution Methods
Tracking the impact of your marketing efforts doesn’t require complex systems. Simple, creative attribution methods often provide clearer insights than elaborate dashboards.
A simple but effective attribution tactic is to use unique contact details—like a dedicated phone number or email address—on specific marketing assets. This creates a clear link between the asset and any resulting inquiries or conversions, helping you directly connect marketing efforts to business outcomes.
For B2B tech companies, connecting marketing touchpoints to sales conversations is particularly crucial. Consider these simple attribution methods:
- Create unique landing pages for different marketing initiatives
- Use UTM parameters consistently across all digital campaigns
- Ask new customers how they found you and document their responses
- Track content consumption before demo requests
These simple tactics can reveal which marketing channels drive the most qualified leads. Over time, these insights help justify where to invest more budget, especially when specific touchpoints consistently correlate with higher conversion rates.
When presenting these insights to leadership, focus on telling a clear story about how marketing activities influence revenue generation, not just activity metrics.
Conclusion
Implementing AI in your B2B marketing isn’t about replacing human intelligence. It’s about enhancing it. By following the four steps we’ve explored — establishing meaningful metrics, collecting the correct data, improving human decisions, and implementing simple attribution methods — you create a foundation where AI can drive results rather than just cut costs.
Always prioritize effectiveness before efficiency. When your marketing strategy is focused on driving tangible results first, any efficiency gains from AI implementation become more valuable, sustainable, and aligned with business growth.
From my conversation with Mike, I’ve come to appreciate that AI isn’t a marketing strategy—it’s a powerful tool within a thoughtful strategy. The most successful B2B tech companies aren’t asking “How can we use AI?” but “How can AI help us better serve our customers and grow our business?”
Ready to dive deeper into these concepts? Listen to my conversation with Mike Moran on the Marketers in Demand’s Content Logistics podcast for more insights on implementing AI in your marketing strategy.
FAQs:
Q1: How much should a small B2B marketing team invest in AI tools?
Start with 5-10% of your current marketing technology budget, focusing first on tools that improve your analytical capabilities rather than content generation. Choose platforms that address your most critical effectiveness gaps and master them before expanding. Many successful B2B companies begin with a single AI tool that enhances their marketing strategy, then gradually increase investment as they demonstrate positive results. Remember that the people using the tools often require more investment (in training and process development) than the technology itself.
Q2: What are the most common mistakes companies make when implementing AI in their marketing?
The biggest implementation mistakes include starting without a clear problem statement, neglecting the AI training period, underinvesting human guidance, and failing to establish performance baselines before adoption. Many companies also fall into the trap of treating AI as a strategy rather than a tool to execute strategy. Another common error is implementing AI tools in isolation from existing workflows, creating disconnected systems that ultimately reduce team productivity despite seeming efficient on paper.
Q3: How can I convince my leadership team to focus on effectiveness before efficiency?
Frame the conversation around risk mitigation and competitive advantage. Prepare a simple ROI comparison showing two scenarios: optimizing marketing effectiveness first versus scaling potentially ineffective tactics. Support your case with concrete examples from competitors who have successfully followed this approach. If met with resistance, propose a small pilot program with clear success metrics demonstrating the effectiveness-first approach in one marketing area.
Q4: Which marketing functions should we automate with AI first?
Focus on augmenting rather than replacing marketing functions, starting with data-intensive tasks, pattern-recognition-heavy, and repetitive yet still require strategicthat are heavy on pattern recognition oversight. For most B2B tech companies, this means beginning with marketing analysis rather than content creation. Consider tools that help identify patterns in customer behavior, optimize existing content, or enhance personalization capabilities. Data processing, audience segmentation, and performance analytics deliver the most immediate value while building team confidence. Consider implementing a growth marketing strategy for a comprehensive approach to scaling effectively.
Q5: How do we maintain brand authenticity while using AI for marketing?
Document your brand voice with specific language examples that align with your brand values, not just vague descriptors. Establish a consistent review workflow where AI-augmented content is regularly evaluated against these standards. Implement a hybrid approach: use AI for research, outlining, and first drafts, while having human marketers handle final editing and approval. Be transparent with your audience about your thoughtful use of technology – today’s B2B buyers appreciate honesty, and acknowledging AI use can actually build trust.
Q6: How often should we re-evaluate our AI implementation strategy?
Review your AI implementation strategy quarterly at minimum, focusing on three questions: Are we seeing measurable improvements in effectiveness Is our team successfully integrating AI tools into workflows? Have new capabilities emerged that better address our challenges? Create a simple AI scorecard tracking these elements over time. The most successful B2B tech companies treat AI implementation as an ongoing capability development process rather than a one-time project, allowing them to adapt as their needs and the technology evolve.